Predicting users’ future interests on social networks: A reference framework
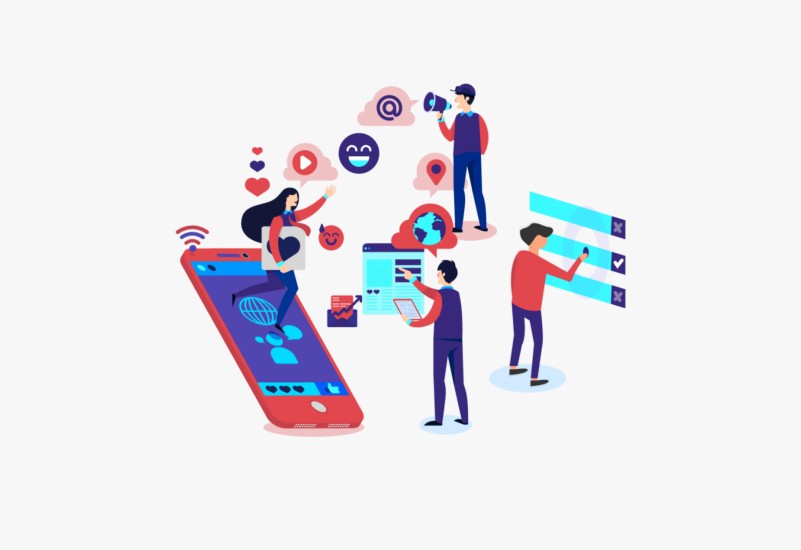
In this research, we propose a new approach to understanding and predicting future interests of users on social networks by analyzing their past activities and interactions. Previously, research primarily focused on current interests, but we aim to forecast interests that users may develop in the future. We categorized the data used for predicting interests into four main types: information based on the user, topics the user has engaged with, explicit interactions between the user and specific topics, and the influence of the user's friends.
We also explored how the timing of past activities influences predictions. Our approach treated the task as a ranking problem, where different types of data were evaluated to determine their effectiveness in predicting future interests.
Based on a real-world dataset from Twitter, we found that the most powerful predictor of a user's future interests was their past direct engagements with topics. Removing this data significantly reduced prediction accuracy. Other strong predictors included the popularity and freshness of topics, particularly for less active users. Although temporal data generally improved predictions, specific time-related topic data didn't have a substantial impact.
Our findings suggest that a deep understanding of a user’s past interactions and topic characteristics is crucial for effectively predicting future interests. This insight could enhance the personalization of content and services on social platforms.Zarrinkalam, F., Alizadeh Noughabi, H., Noorian,Z., Fani, H., & Bagheri, E. (2024). Predicting users’ future interests on social networks: A reference framework. Information Processing & Management, 61(5), 103765.