Out of the echo chamber
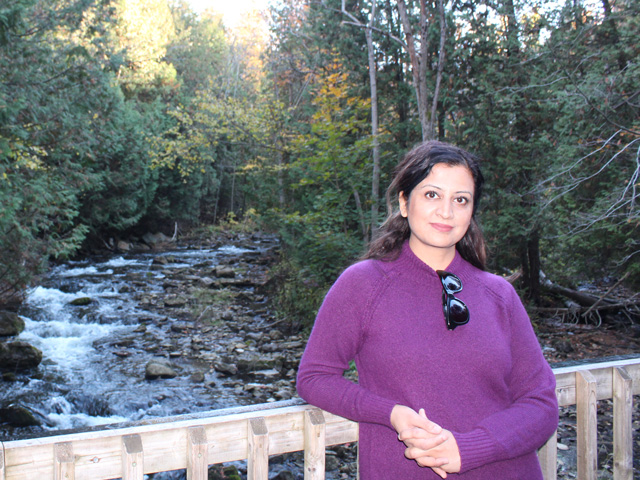
Shaina Raza
Online news outlets make it easy to access a variety of news sources from around the world. At the same time, the sheer amount of information available can be overwhelming. No-one has the time to scour every preferred source and also explore potentially interesting novel sources. This is why news recommender systems (NRS) exist—relying on the logic of artificial intelligence (AI) algorithms, they help users access content they care about. They also serve commercial purposes, such as attracting readership and generating revenue through click-through-rate, by attempting to maximize a user’s engagement with a particular news site.
But in providing news recommendations highly aligned with past preferences, recommender systems may also cause a decrease in the diversity of information a reader accesses. Accuracy grows but variety declines. This can create a number of problems, ranging from boredom to getting trapped inside an echo chamber, which exists when a reader only gets news reflecting their opinions. While a reader might initially be curious about a particular topic or position, the AI algorithm is designed to gradually narrow news options until most or all recommendations reflect and reinforce a singular viewpoint.
With the support of supervisor Dr. Chen (Cherie) Ding, computer science PhD student Shaina Raza conducted research aimed at providing news recommendations to readers with a balanced objective, mixing accuracy (based on past behaviour) with reasonably diversified recommendations. This approach addresses both the boredom issue and the potential of getting trapped inside filter bubbles. Raza’s recently published paper (external link) demonstrates the effectiveness of a new model that combines accuracy and diversity in a single optimization framework.
“The findings of this research are novel and show that the user satisfaction is not associated with the high algorithmic accuracy,” says Raza. “The state-of-the-art news recommender systems usually associate user satisfaction with a high accuracy measure. But we have found that accuracy does not guarantee a better user experience. While the accuracy must be high, it must also be balanced with reasonable diversity. Our novel news recommender system intelligently trades off between the contradictory aspects of accuracy and diversity.”
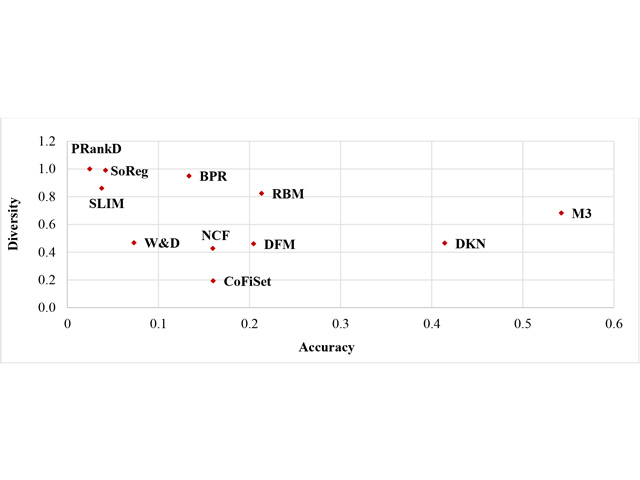
The negative correlation between accuracy and diversity. We show in this figure that diversity and accuracy have negative correlation. When accuracy increases, the diversity decreases and vice versa. The state-of-the-art is shown with diamond shaped dots with their names, and our proposed model is shown by name M3. We can see that in this result, our proposed model gets high accuracy and a reasonable diversity (as we aimed for).
With Raza’s proposed idea, readers will get the personalized and up-to-date news recommendations they want. They will also get recommendations that expose them to counter-attitudinal behaviour.
Big names in Internet services like Google and Microsoft rely heavily on providing the highest accuracy from their recommender system. Many readers are unaware that these services use their browsing history, age, gender, location and other data to select the stories that come their way, resulting in a flood of articles that support their current opinions. Raza’s model has the potential to liberate readers from filter bubbles, broaden perspectives, and counteract disinformation, misinformation and the wide-scale proliferation of fake news.
“A clear example of this is the spread of anti-vaccination misinformation,” says Raza. “The implications of such news are seen in the anti-vaccine movements hindering the global fight against COVID-19. It’s critically important for readers to get out of filter bubbles, and news recommender systems have an important role to play in that.”
Raza’s research shows that state-of-the-art accuracy is not all that matters to readers and, therefore, not the only measure of NRS quality. With her model’s tradeoff scores higher than those of dominant models, she illustrates that balance and diversity can engage readers, expand civic dialogue, and elevate commercial profit.
This research has been partially supported by an NSERC grant.