Research
Geographic Collaboration
The research focuses on design and use of technologies to enable groups to work productively with geospatial information, to develop concepts, methods, frameworks, system architecture and models, and investigate collaborative awareness, concurrent control, collaborative behavior modeling and other related key issues, which are required to develop multi-user, asynchronous or real-time collaborative geospatial environments, e.g., what you see is what I see (WYSIWIS), for spatial decision support and problem solving.

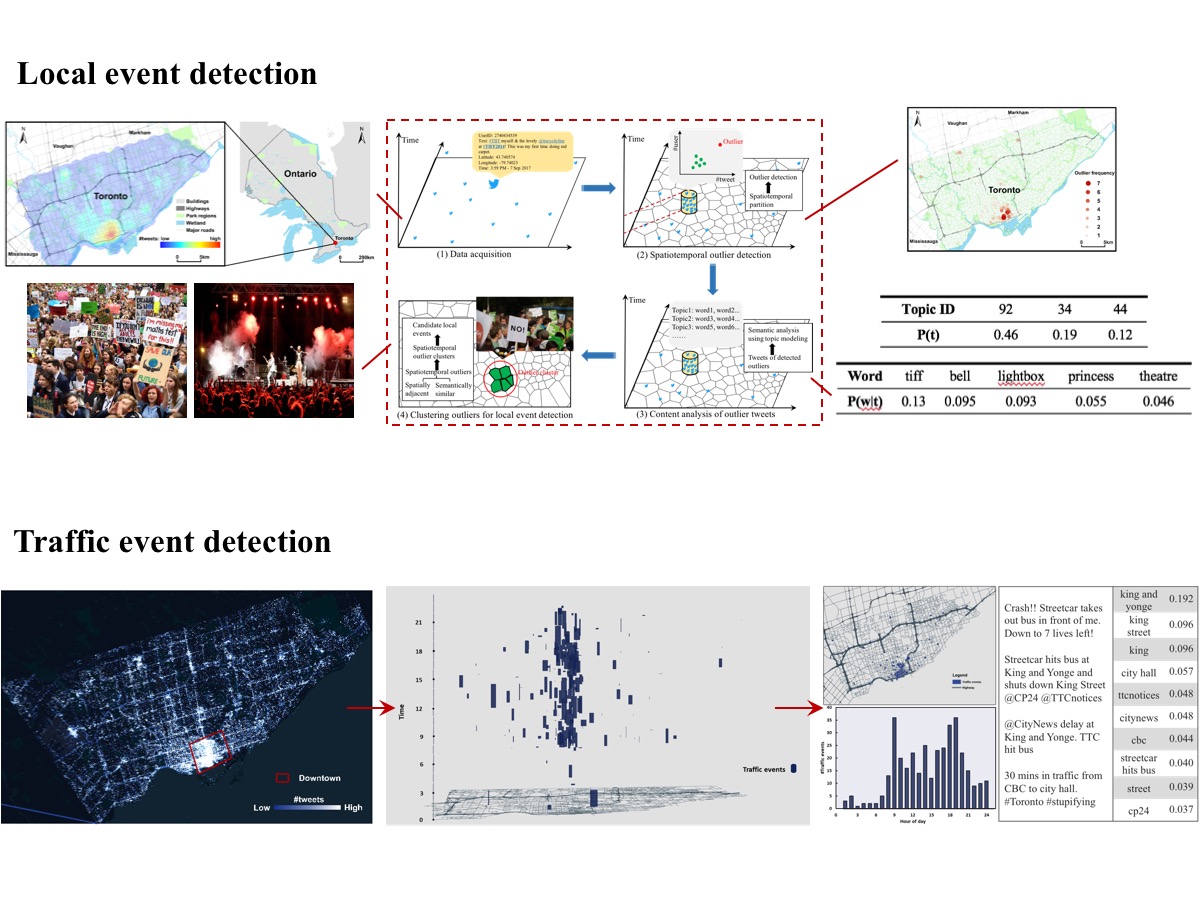
Geospatial Event Detection
The research develops methods and algorithms for detecting geospatial events using big data such as geosocial media data, trajectory data, sensors data and other crowsoured data to form an event-driven service-oriented GIS infrastructure (e.g., Sense & Response GIS) and applications, which takes events from different sources including sensors, web services, applications and databases; processes spatial and non-spatial events together with external geographic data; and notifies event subscribers according to their subscriptions (possibly “spatially-enabled”), who in turn invoke proper actions.
Geosocial Media Data Analytics
The research focuses on data modeling and spatio-temporal analysis of big geosocial media data to reveal relationships in data, detect events and patterns (e.g., human mobility patterns), to extract location intellience, and to develop predictive models in geosocial data.


Urban Solar Energy Modeling
This research develops easy-to-use, integrated urban solar energy modeling, mapping and demonstration techniques to provide the capability for non-expert users to easily model solar energy potential on residential/industrial building rooftops and other urban infrastructure, and link it to energy usage data for better public awareness, understanding of solar potential and engagement into current feed-in-tariff programs.
Road Surface Monitoring using Smartphone Sensors
Smartphone-based sensing has become an important supplemental technique in detecting road surface anomalies since they are equipped by different kinds of physical sensors and virtual sensors. This research focuses on the development of methods and algorithms for detecting road surface anomalies based on crowdsourced sensors data. The large amount of detected road surface anomalies can then be integrated and analyzed to identify potholes, cracks and bumps on roads in near real time, as well as evaluate road surface conditions.
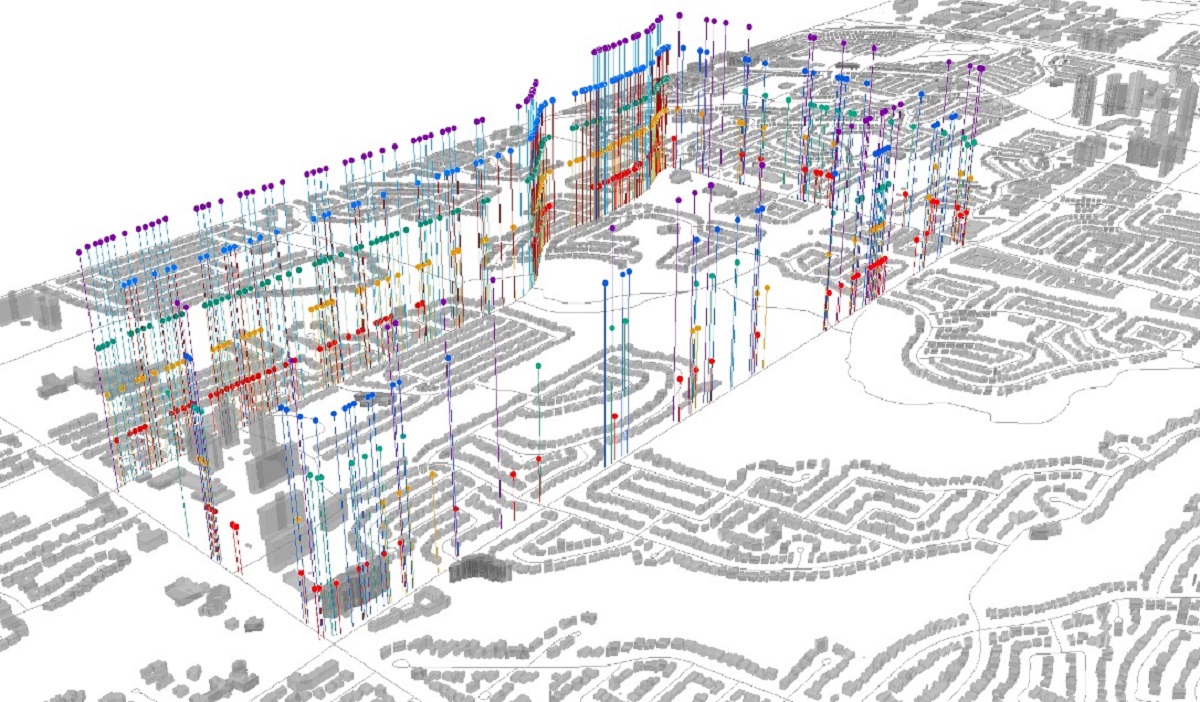